How does Retrieval Augmented Generation Work?
It acts as a link to external sources provided by the model users. Any works that are cited can then be placed as footnotes within the model’s generated content for the users to inspect and investigate further to validate the Gen AI’s response. Because of the added layer of accuracy, models can provide much more in-depth answers resulting in less confusion and fewer hallucinations. Surprisingly, RAGs also result in models that are both easier to set up and less expensive to maintain and provide sources for. These can be interconnected with multiple datasets using different data types and mediums, and have already garnered sufficient attention from multiple tech giants for the potential they offer.
As an advanced AWS partner, we bring unparalleled expertise to architect, deploy, and optimize cloud solutions tailored to your unique needs.
How Retrieval-Augmented Generation Works
A couple of models that covered this functionality previously existed. Retrieval-based models are built to retrieve responses or information from a predefined set of responses based on the input query. These models are great for archive models as they excel at drawing relevant information from a repository of existing knowledge and responses with little need to restructure anything. Comparatively, generative models can construct new content over having to rely strictly on predetermined responses. Typically built on neural networks trained on massive datasets to comprehend better and imitate human text.
Retrieval-augmented generation models, by comparison, are an amalgamation of these two model types. This approach integrates the former model’s retrieval mechanism with the latter’s natural language component. By extension, this model is comparatively flexible, having both a physical library of content to access to provide tangible and related facts to bolster its answer with the capacity to restructure it to best fit the user’s prompt.
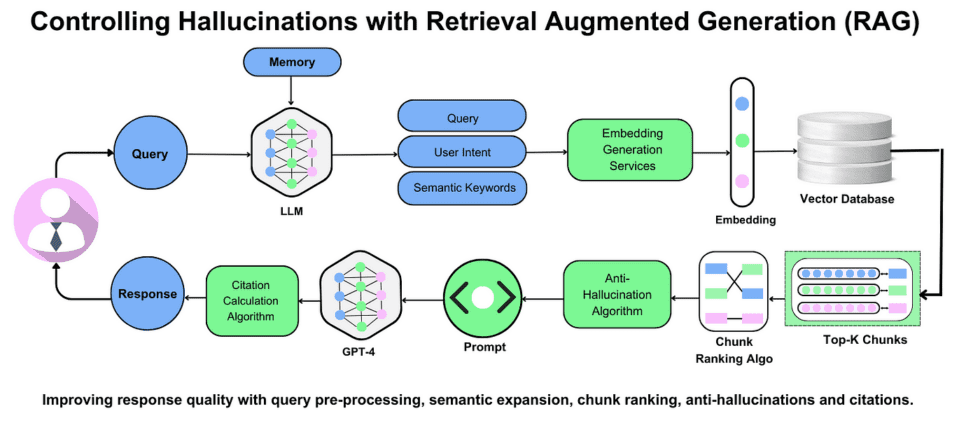
Conclusion
People’s most significant concern with Gen AI is plagiarism and an inability to properly cite sources if any content produced by a model is used. Despite the recent developments made to Gen AI models, hallucinations and other errors are still common in the outputs. By more closely integrating sources and source repositories into Gen AI models, this can sharply increase the accuracy models have and provide tangible sources they draw their conclusions and outputs from.
Get Started Today!
At AllCode, our mission is to leverage our unique skillset and expertise to deliver innovative, top-tier software solutions that empower businesses to thrive in our world’s rapidly-evolving technological landscape.
Work with an expert. Work with AllCode
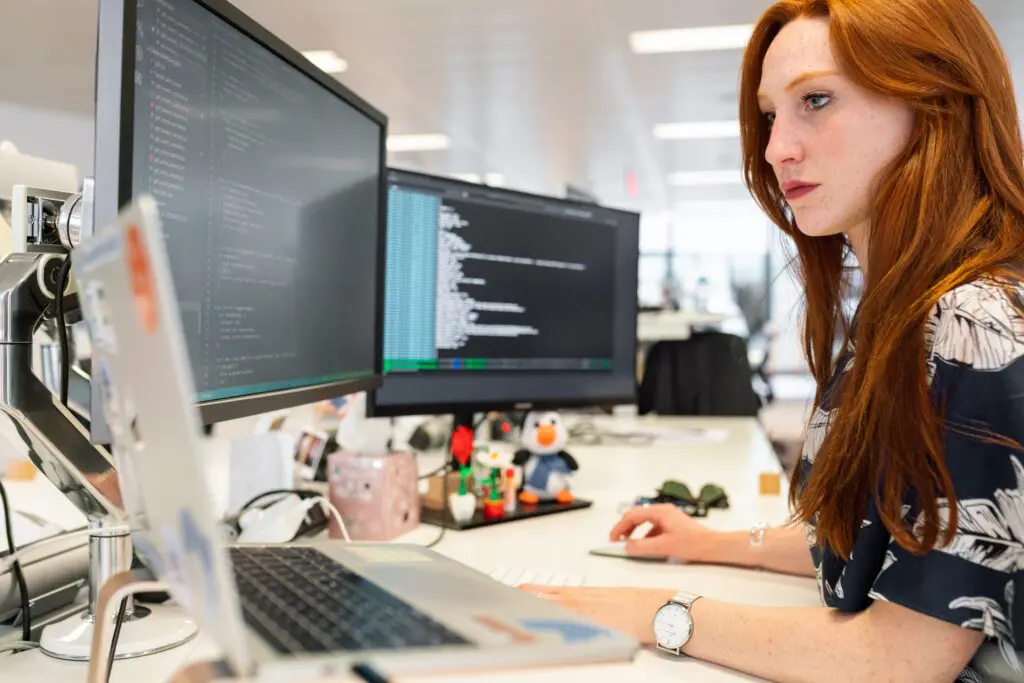