Understanding Predictive Maintenence
Predictive maintenance involves forecasting potential issues in machinery or equipment before they lead to downtime or failure. Traditional methods relied on historical data and statistical models to predict when maintenance is required. However, the rise of AI has introduced more sophisticated approaches, such as machine learning algorithms and, more recently, generative models.
The Emergence of Generative Models
Generative models, a subset of machine learning models, have gained prominence for their ability to generate new data samples that resemble the training data. While they have been widely used in image and text generation, their application in predictive maintenance is a relatively recent development.
As an advanced AWS partner, we bring unparalleled expertise to architect, deploy, and optimize cloud solutions tailored to your unique needs.
Generative Models in Predictive Maintenance
Generative models bring a new dimension to predictive maintenance by simulating and generating possible failure scenarios. Unlike traditional models that focus on predicting when maintenance is needed based on historical patterns, generative models allow for the creation of synthetic data representing potential failure modes.
Enhancing Anomaly Detection
Anomaly detection is a crucial aspect of predictive maintenance. Generative models excel in identifying anomalies by learning the normal patterns from the training data and flagging any deviations as potential issues. This enhances the accuracy of maintenance predictions and reduces false positives, enabling organizations to focus their resources on addressing genuine concerns.
Optimizing Maintenance Strategies
The real power of generative models lies in their ability to optimize maintenance strategies. By generating diverse failure scenarios, these models provide insights into the possible impacts of different maintenance approaches. Organizations can then tailor their strategies based on the most probable failure modes, leading to more efficient resource allocation and reduced downtime.
Integration with IoT Solutions
The synergy between generative models and IoT solutions is a game-changer in the realm of predictive maintenance. IoT devices continuously collect vast amounts of data from connected assets. Generative models can leverage this data to create realistic failure scenarios, taking into account the complex interplay of variables in an IoT ecosystem.
Reducing Unplanned Downtime
Unplanned downtime can have severe financial implications for businesses. Generative models, by predicting potential failure modes accurately, empower organizations to implement proactive maintenance measures. This not only minimizes unplanned downtime but also extends the lifespan of assets, resulting in substantial cost savings.
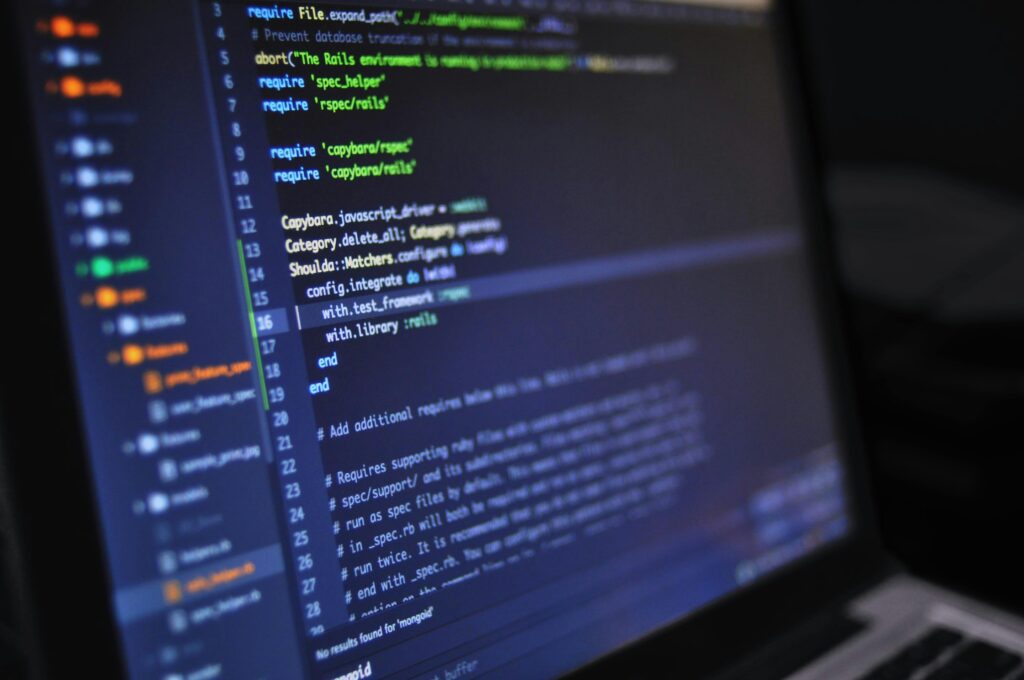
Challenges and Considerations
While the potential benefits of generative models in predictive maintenance are evident, there are challenges to overcome. Ensuring the reliability of generated scenarios, addressing data quality issues, and managing the computational complexity of these models are areas that require careful consideration.
Case Studies: Real-world Applications
Examining real-world applications provides concrete examples of the effectiveness of generative models in predictive maintenance. Highlighting successful implementations across various industries underscores the versatility and applicability of these models in optimizing maintenance strategies.
Considerations
In conclusion, the integration of generative models in AI-driven predictive maintenance for IoT solutions marks a significant leap forward in asset management. The ability to simulate diverse failure scenarios and optimize maintenance strategies enhances the accuracy and efficiency of maintenance practices. As organizations continue to embrace the potential of generative models, the landscape of predictive maintenance is poised for further innovation and improvement.
Get Started Today!
At AllCode, our mission is to leverage our unique skillset and expertise to deliver innovative, top-tier software solutions that empower businesses to thrive in our world’s rapidly-evolving technological landscape.
Work with an expert. Work with AllCode
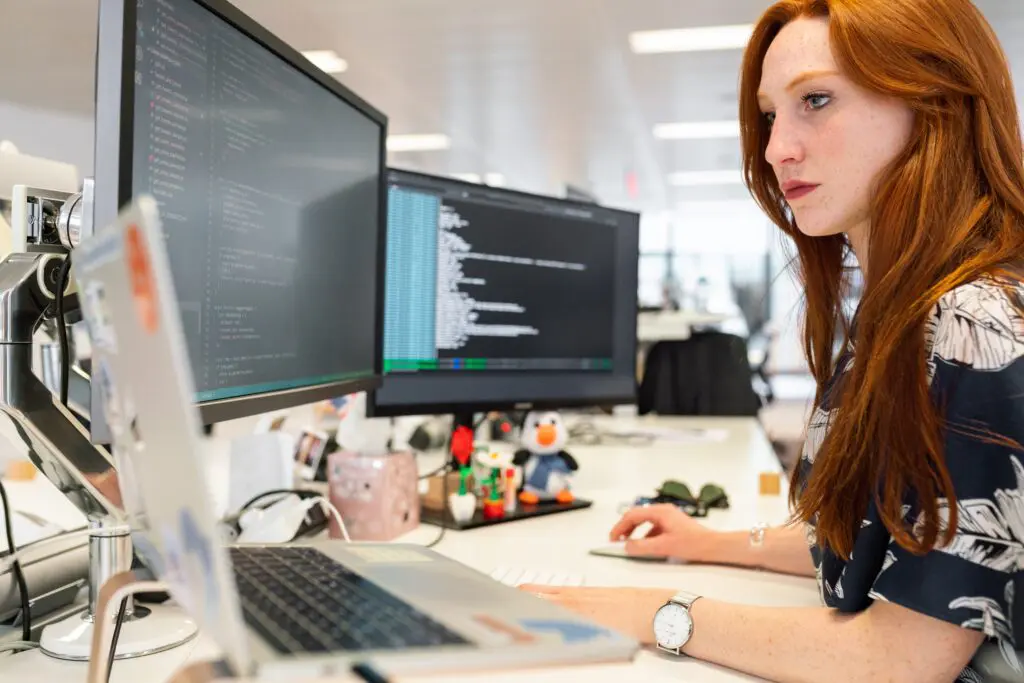