Predictive Maintenance and Internet of Things
Internet of Things devices can be a headache to fix. Traditionally, maintenance of IoT devices has been reactive, often responding to issues only after they occur. With so many different device types utilizing different software, different firmware, and potentially being placed in vastly different environments, there are more than enough hurdles for repair crews to worry about before even reaching security threats. Predictive maintenance, powered by Generative AI, transforms this approach by leveraging data analytics, machine learning, and predictive modeling to anticipate potential problems before they impact device performance.
Text AWS to (415) 223-9212
Text us and join the 700+ developers that have chosen to opt-in to receive the latest AWS insights directly to their phone. Don’t worry, we’ll only text you 1-2 times a month and won’t send you any promotional campaigns - just great content!
Predictive AI Workflow
The process is fairly simple on paper. With how much data IoT devices must cycle, there is more than enough data to give a predictive model material to plan with. Assuming all data sources are correctly connected to the model, the process is as follows:
1. Data Collection
IoT devices can potentially process large quantities of data per day, including sensor readings, historical performance metrics, and environmental conditions. This will be the core material Generative AI will work with to anticipate potential faults and malfunctions.
2. Training Generative AI Models
Train Generative AI models using historical data to establish normal behavior patterns and identify anomalies. Alternatively, set up the Generative AI models to simulate different failure scenarios, allowing for a comprehensive analysis of potential failure modes and the likelihood of what their primary causes are. This empowers maintenance teams to develop targeted strategies for prevention and mitigation.
3. Real-Time Monitoring
Implement real-time monitoring of IoT devices using Generative AI to continuously analyze incoming data streams. By analyzing various parameters such as temperature, vibration, and power consumption, the AI models can assess the health of the devices, map current trajectories of existing data points, and accurately predict impending issues. Integrate automated alert systems to notify maintenance teams of predicted issues and provide recommendations for preventive actions.
4. Anomaly Detection
Generative AI models excel in identifying anomalies within large datasets generated by IoT devices. With proper training, a model can detect anomalies or deviations from established patterns and trigger user alerts for potential maintenance requirements. By establishing baseline patterns of normal behavior, these models can detect deviations that may indicate potential issues.
5. Predictive Analytics
Utilize predictive analytics to forecast when specific components or systems are likely to fail, allowing for proactive maintenance scheduling. By leveraging historical data, Generative AI can predict when specific components of an IoT device are likely to fail. This proactive approach enables scheduled maintenance, preventing unexpected downtime.
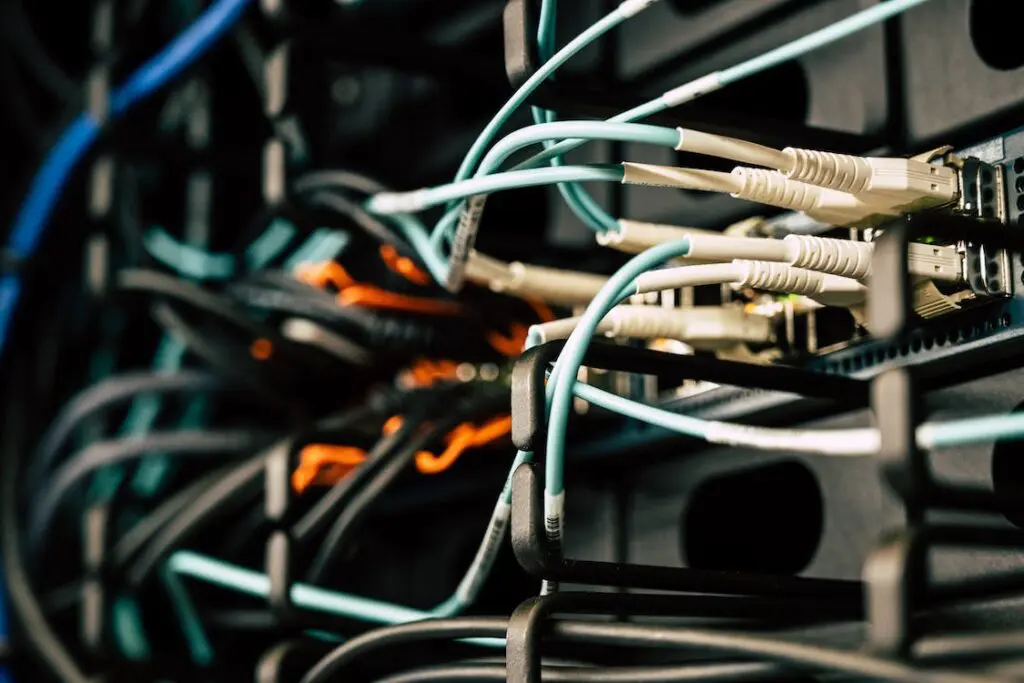
Benefits of Using Generative AI for IoT Maintenance
Increased Reliability
Generative AI enables early detection of potential issues, reducing the likelihood of device failures and enhancing overall system reliability.
Reduced Downtime
Proactive maintenance based on Generative AI predictions minimizes unplanned downtime, ensuring continuous operation of IoT systems.
Cost Savings
By preventing failures and optimizing maintenance schedules, organizations can achieve significant cost savings in terms of repairs, replacements, and operational disruptions.
Improved Efficiency
On occasion, human error will still allow small problems to slip through the cracks that will result in these problems in the first place. Generative AI helps alleviate this by automating the monitoring and analysis process so maintenance teams can focus efforts on strategic planning and execution rather than reactive responses.
Count on our support to guide your success
Use Cases
Wind Turbines
As much as wind turbines have proven their worth, they are no less high-maintenance infrastructure. Constantly bombarded by the elements, maintaining these turbines is critical to maintaining constant power intake. By predicting potential issues in the rotating blades or internal components, maintenance teams can schedule repairs during optimal conditions, maximizing operational efficiency.
Manufacturing Equipment
Manufacturing plants are host to a wide variety of machinery. To keep production moving, everything must be functional and prepared to move the supply chain along. Predictive maintenance based on AI insights ensures that production lines operate smoothly, minimizing downtime and enhancing productivity.
Increasing IoT Resilience
The integration of Generative AI in predictive maintenance for IoT devices is an evolving field. As AI models become more sophisticated, the accuracy of predictions will improve, allowing for even more precise maintenance strategies. The future holds the promise of fully autonomous predictive maintenance systems that seamlessly adapt to the dynamic nature of IoT environments.
It has become the cornerstone of a transformative shift in how we approach maintenance in the IoT era. By predicting and preventing issues before they escalate, Generative AI ensures that IoT ecosystems operate with unprecedented reliability, efficiency, and resilience. How Internet of Things devices inhabit our infrastructure will gradually become more reliable and less prone to faults with the increased introduction of Generative AI.