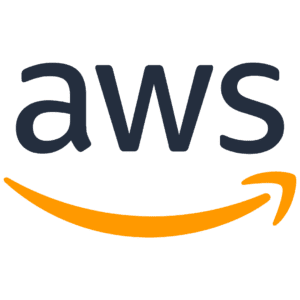
AWS SageMaker
Benefits of SageMaker
- Enhance the usability of ML
If the tools that are accessible to innovate with machine learning include integrated development environments for data scientists and visual interfaces that don’t require any coding for business analysts, then more people will be able to innovate with machine learning (ML).
- Prepare data on a large scale.
Both structured (table data) and unstructured (pictures, video, and audio) information must be gathered, categorized, and processed in order to aid in machine learning applications.
- Accelerate the emergence of novel machine learning approaches.
Training can be cut down from hours to minutes or even seconds with the right infrastructure. You can tenfold the productivity of your team using custom tools.
- Streamline the Machine Learning (ML) lifecycle.
Create, train, deploy, and manage models in a distributed environment at scale by standardising and automating MLOps procedures throughout your organisation.
The automation of the entire machine learning lifecycle is made possible through Amazon SageMaker Pipelines. This feature encompasses data preparation, training, and deployment, allowing for seamless end-to-end workflows. To ensure ongoing model quality, Amazon SageMaker Model Monitor enables teams to monitor and receive timely notifications of any issues that may arise. This proactive approach empowers teams to take immediate action, ensuring optimal performance and accuracy.
For effortless deployment of ML models, Amazon SageMaker Serverless Inference eliminates the need for managing servers or clusters. Additionally, SageMaker Inference Recommender saves teams the trouble of building their own testing infrastructure, as it assists in selecting the ideal deployment configuration and conducting load tests for optimal inference performance.
How it Works
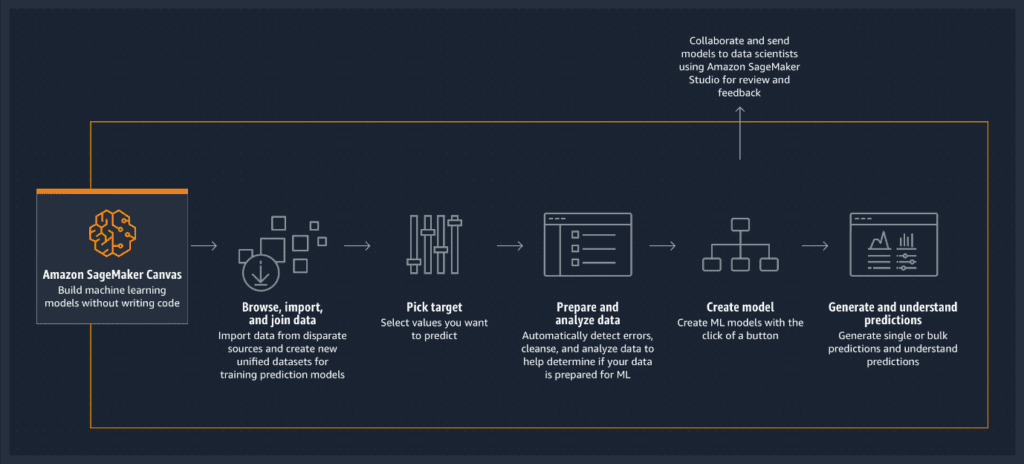
Image sourced from Amazon Web Services
Amazon SageMaker Data Wrangler
Amazon SageMaker Data Wrangler reduces the time it takes to prepare data for machine learning (ML) from weeks to minutes. A single visual interface may be used to accomplish all of the work needed in data preparation and feature engineering, from selection through exploration and visualisation, using SageMaker Data Wrangler. SageMaker Data Wrangler’s data selection tool makes it easy to import data from multiple sources.No need to create any code to normalize, convert, or combine features using SageMaker Data Wrangler’s more than 300 built-in data transformations Amazon SageMaker Studio’s integrated development environment (IDE) makes it simple to evaluate and analyse these alterations to ensure that they are done exactly as you intended. Using Amazon SageMaker Pipelines, you can construct completely automated machine learning processes, which you can then save in the Amazon SageMaker Feature Store for future use.
- Quickly prepare data for ML
Choosing and querying data only takes a few mouse clicks.
As well as Amazon SageMaker Data Wrangler, AWS Lake Formation, and Amazon S3, Amazon Athena, Amazon Redshift, and AWS SageMaker Feature Store, AWS SageMaker Data Wrangler also provides data selection choices for AWS Lake Formation. A wide range of data sources, including CSV files, Parquet files, and database tables, can be accessed directly by SageMaker.
To ensure the highest quality models, the Amazon SageMaker Debugger plays a crucial role by capturing measurements and characterizing training jobs in real-time. This enables teams to identify and address any issues before deploying the models into production. Additionally, by leveraging existing resources, SageMaker allows for substantial cost savings of up to 90% on training expenses. The platform achieves this through the exploration of billions of algorithm parameters, significantly improving model accuracy while saving weeks of valuable time and effort.
- Transform data quickly and easily
A single line of code is not required to change your data with SageMaker Data Wrangler’s 300+ pre-configured transformations. With a single click, you can convert a text field column to a numeric column in Python, SQL, and Pandas.
With Amazon SageMaker, MLOps teams can enjoy accelerated training times thanks to the SageMaker Training Compiler, which utilizes GPUs to boost performance by up to 50%. The process is streamlined, requiring only the specification of data location and desired SageMaker instances to get started promptly. Furthermore, SageMaker simplifies the delivery of Amazon-distributed training, making it effortless for teams to leverage the power of distributed computing.
- Visualize your data to learn more
Pre-configured visualisation templates are available in SageMaker Data Wrangler to help you better comprehend your data. Every type of graph, from histograms to bar charts, can be found here. To develop and modify visualisations, you don’t need to write any code.
- Get ML model accuracy quickly
Diagnostics based on ML data prepared more quickly
SageMaker Data Wrangler assists you in detecting discrepancies in your data preparation routines before models are sent into production. You may rapidly examine the veracity of the data you’ve provided and determine whether additional feature engineering is required to boost performance.
- One click from prep to production
Streamline the pre-processing of ML data
With a single click, you may save your data preparation technique as a notebook or script. SageMaker Data Wrangler integrates with Amazon SageMaker Pipelines to facilitate model deployment and management. The Amazon SageMaker Feature Store publishes features that your team can use in their own models and analyses, which in turn makes them available to other teams.
Download list of all AWS Services PDF
Features
- Transparency
In order to increase model quality, Amazon SageMaker Clarify automatically detects biases during data preparation and after training. SageMaker Clarify’s model explainability reports help stakeholders better understand how and why models make predictions.
- Protection and Confidentiality
Amazon SageMaker provides a completely secure machine learning environment from the start, making it easy to get up and running quickly. Security elements can be used to ensure compliance with a wide range of industrial laws.
- Data Marking and Labeling
Create accurate training datasets with Amazon SageMaker Ground Truth Plus without having to build or manage labelling teams on-premises. Amazon SageMaker Ground Truth Plus provides a skilled workforce and a centralized database to control the workflows.
- Featured Retailer
Amazon SageMaker Feature Store provides ML features in both real-time and batch modes through its ML feature set. During the training and inference phases, features can be safely stored, discovered, and shared. This saves a lot of time during development.
- Amazon’s Data Processing on a Massive Scale SageMaker
Simplified data processing is now possible because to the scalability, dependability, and ease of use of SageMaker. SageMaker Processing allows you to connect to an existing storage system, provision resources for your job’s execution, store output to a persistent storage location, and collect logs and metrics, all of which are possible.
- Machine Learning with No Coding
Businesses can use Amazon SageMaker Canvas instead of writing code or having prior understanding of machine learning to develop machine learning models and make accurate predictions. You can also publish your results, explain and analyse your models, and share your models with others using this software.
- Free Machine Learning Environment
Amazon AWS’s SageMaker Studio Lab offers a no-configuration free development environment for building machine learning models. An open-source Jupyter Notebook linked to GitHub is included with Amazon SageMaker Studio Lab, along with 15 GB of dedicated storage.
- Creating Jupyter Notebooks is as simple as clicking a button.
Using Amazon SageMaker Studio Notebooks, you have one-click access to Jupyter notebooks and can quickly scale up or down the computing resources that are available. Your colleagues can access the same notebook that you have saved in the same spot when you share it with a single click.
- Algorithms Pre-installed
With over 15 built-in algorithms, Amazon SageMaker delivers a wide range of pre-built container images that may be used to train and do inference.
- Both pre-built and open-source models are options.
It’s easy to get started with machine learning using Amazon SageMaker JumpStart, which uses pre-built solutions that can be deployed in a few clicks. With a single click, more than 150 widely used open-source models may be deployed and fine-tuned.
- AutoML
Automated machine learning models are trained and tuned using your own data, all under your total and complete control. Next, you have the option to either publish the model to the production environment with a single click or work on it to improve its accuracy.
- Optimized for the most popular frameworks
Many of the most popular deep learning frameworks are supported by Amazon SageMaker, including TensorFlow, Apache MXNet, PyTorch, and more. Using the most recent version, frameworks are constantly up-to-date and optimized for AWS performance. As long as you’re using the built-in containers, these frameworks don’t require any further configuration.
- Local Mode
Amazon SageMaker makes it feasible to conduct local testing and prototyping. From the GitHub repository, you can get Apache MXNet and TensorFlow Docker images used in SageMaker for your own usage. You can use the Python SDK and these containers to test scripts before deploying them to training or hosting environments.
Need help on AWS?
AWS Partners, such as AllCode, are trusted and recommended by Amazon Web Services to help you deliver with confidence. AllCode employs the same mission-critical best practices and services that power Amazon’s monstrous ecommerce platform.
- Amazon’s Reinforcement Learning System
Amazon SageMaker is a powerful platform that offers a comprehensive suite of tools to simplify the development, training, and deployment of machine learning (ML) models. With the recent addition of reinforcement learning capabilities, SageMaker incorporates some of the most advanced algorithms from academic literature, ensuring you have access to the latest techniques.
One of the standout features of SageMaker is Amazon SageMaker Experiments, which allows you to track the iterations of your machine learning models. It stores the input parameters, configurations, and results of your experiments, making it easy to compare and analyze the outcomes. SageMaker Studio provides a user-friendly interface to view and compare experiment results, as well as design and execute custom tests. SageMaker is designed to optimize resource utilization and cost-efficiency. By leveraging your existing resources, you can save up to 90% on training costs. SageMaker automatically runs training jobs when additional compute power becomes available and handles disruptions caused by changes in resource availability. With billions of algorithm parameter combinations, SageMaker’s automatic model tuning can significantly enhance your model’s accuracy, saving you valuable time and effort.
To speed up training, SageMaker utilizes the Amazon SageMaker Training Compiler, which can boost training speeds by up to 50% using GPUs. This acceleration is available for popular frameworks like TensorFlow and PyTorch while maintaining compatibility with their standard implementations. Getting started with training is straightforward – simply provide the data location and specify the SageMaker instances you want to use, and you’ll be up and running in no time. After training, SageMaker automatically sends the results to Amazon S3, decommissions the cluster, and prepares for the next iteration.
SageMaker scales effortlessly, allowing you to handle large-scale training and deployment requirements. By employing SageMaker’s distributed training capabilities, you can achieve near-linear scaling efficiency by distributing your data across multiple GPUs. This ensures that you can train complex models efficiently and effectively.
- Management and tracking of experimental results
You may maintain track of your machine learning model iterations with Amazon SageMaker Experiments, which store the input parameters, setups and results of your experiments. SageMaker Studio can be used to view and compare experiment outcomes. In addition, SageMaker Studio lets you design and execute your own tests.
- Runs for debugging and profiling are included.
To ensure the highest quality models, the Amazon SageMaker Debugger plays a crucial role by capturing measurements and characterizing training jobs in real time. This enables teams to identify and address any issues before deploying the models into production. Additionally, by leveraging existing resources, SageMaker allows for substantial cost savings of up to 90% on training expenses. The platform achieves this through the exploration of billions of algorithm parameters, significantly improving model accuracy while saving weeks of valuable time and effort.
- Spot training under supervision
With Amazon SageMaker, you may save up to 90% on training costs by utilizing your existing resources. Once extra compute power is made available, training jobs are automatically run and engineered to withstand disruptions caused by changes in the availability of computing resources. SageMaker makes it easy to deliver Amazon-distributed training. It is possible to achieve near-linear scaling efficiency using SageMaker, which allows you to split your data across multiple GPUs. With fewer than ten lines of code, SageMaker can also help you distribute your model across many GPUs.
In addition to these cost-saving benefits, SageMaker also provides robust support for scalability in ML models. It offers MLOps teams the ability to track model performance in real-time, ensuring that any issues or anomalies are detected promptly. Model Monitor, a feature within SageMaker, continuously and on-schedule monitors changes in data quality, model quality, prediction bias, and feature attribution. This comprehensive monitoring capability allows MLOps teams to receive alerts when specific performance thresholds are exceeded or anomalies are detected.
To further assist in optimizing model performance, SageMaker provides detailed reports and visualizations. These reports offer in-depth analysis of model performance, allowing MLOps teams to identify areas for improvement and make data-driven decisions. With these insights, they can continually enhance their ML models.
- Adaptive Model Tuning on an Automated Basis
By experimenting with billions of algorithm parameters, Amazon SageMaker can increase your model’s accuracy and save you weeks of time and effort. Amazon SageMaker simplifies the model development process by leveraging advanced techniques to enhance model accuracy and save valuable time and effort. With the ability to experiment with billions of algorithm parameters, SageMaker fine-tunes models to achieve higher levels of accuracy, eliminating the need for manual adjustments and reducing the time required for optimization.
One of the key features of SageMaker is its automatic model tuning capability. By leveraging machine learning techniques, the platform intelligently explores and tests various combinations of algorithm parameters to identify the optimal configuration for the model. This automated process eliminates the need for manual trial and error, saving weeks of time that would otherwise be spent on fine-tuning.
Moreover, SageMaker offers a user-friendly interface that caters to both experienced data scientists and professionals who rely on no-code tools. This accessibility allows a diverse range of users to easily work with machine learning models, regardless of their technical background.
To further streamline the model development process, SageMaker integrates seamlessly with DevOps tools. This built-in integration enables continuous delivery of machine learning applications, facilitating a smooth workflow for MLOps teams. By automating the deployment and update processes, SageMaker eliminates the need for manual intervention, reducing the potential for errors and ensuring a more efficient development cycle.
- Compiler for Training
By enhancing the graph and kernel levels, the Amazon SageMaker Training Compiler can speed up training by up to 50 percent thanks to the usage of GPUs. You may speed up training in TensorFlow and PyTorch while still keeping SageMaker’s implementations compatible with TensorFlow and PyTorch.
- Training with a single click
For training, all you have to do is provide the location of the data and which SageMaker instances you’d like to utilize, and you’ll be up and running in a flash. After running the training, SageMaker transmits the findings to Amazon S3, decommissions the cluster and begins the process again.
- Distributed Training
SageMaker makes it easy to deliver Amazon-distributed training. It is possible to achieve near-linear scaling efficiency using SageMaker, which allows you to split your data across multiple GPUs. With fewer than ten lines of code, SageMaker can also help you distribute your model across many GPUs.
- CI/CD
Amazon SageMaker Pipelines is a comprehensive solution that encompasses all stages of the model lifecycle, including data preparation, training, and deployment. With SageMaker Model Monitor, you can continuously monitor the quality of your models and receive immediate notifications if any issues arise, enabling you to take prompt corrective actions. This ensures that your models consistently deliver accurate results and maintain high performance.
- Models Must Be Constantly Monitored
Reinforcement learning has been incorporated into Amazon SageMaker’s repertoire of learning capabilities. With its inclusion, MLOps teams can now leverage some of the most recent and highly effective algorithms from academic literature. These algorithms are seamlessly integrated into the SageMaker platform, providing users with a comprehensive set of tools to enhance their machine learning endeavors.
One of the standout features of Amazon SageMaker is its ability to facilitate the tracking of machine learning model iterations through Amazon SageMaker Experiments. This feature enables users to store and manage input parameters, configurations, and results of their experiments. To conveniently view and compare experiment outcomes, MLOps teams can utilize the intuitive interface of SageMaker Studio. Additionally, SageMaker Studio empowers users to design and execute their own tests, granting them greater control over the experimentation process.
One of the notable benefits of utilizing Amazon SageMaker is its ability to optimize resource allocation and minimize training costs. By leveraging existing resources, users can save up to 90% on training expenses. SageMaker automatically runs training jobs when additional compute power becomes available and is engineered to withstand disruptions caused by changes in computing resource availability, ensuring a seamless and cost-effective training experience.
The Amazon SageMaker Debugger plays a crucial role in ensuring the quality of machine learning models before they are deployed into production. By capturing measurements and providing real-time insights into the characteristics of training jobs, this feature enables MLOps teams to proactively identify and address any issues that may arise during the training process.
AWS Service Business Continuity Plan
Thousands of businesses are lose an unprecedented amount of money every quarter - don’t let yours! Protect your AWS services with this FREE AWS Business Continuity Plan. Learn More
• Inferences based on serverless architecture
If you utilize Amazon SageMaker Serverless Inference (preview), you don’t have to bother about servers or clusters while deploying ML models. With the help of Amazon SageMaker, it is possible to scale and shut down compute capacity automatically.
• Inference Adviser
SageMaker Inference Recommender makes it unnecessary for you to construct your own testing infrastructure and execute load tests to optimize inference speed. Alternatively, you can run a fully-managed load test on any of the instance types you choose for your model deployment.
• Incorporation of Kubernetes
Use SageMaker Inference Recommender to pick the ideal deployment configuration and run load tests for optimal inference performance without having to develop your own testing infrastructure. Any of the instance types that you employ for your model deployment can be subjected to a fully-managed load test instead.
• The Endpoints of Multiple Models
To run a large number of custom machine learning models at a low cost and with minimal effort, Amazon SageMaker is a great option. Using the SageMaker Multi-Model endpoints, a single SageMaker endpoint may be used to install and serve many SageMaker models.
• Inference Pipelines
For batch and real-time inference, Amazon SageMaker’s Inference Pipelines can be utilized to transmit and process raw input data. Inference Pipelines can be used to develop and distribute feature-data processing and feature engineering pipelines.
• Any Device Can Run Models
At the same time, Amazon SageMaker Neo may be used to train and deploy machine learning models on-the-fly or in the cloud. A trained model’s performance can be boosted by up to two times utilising SageMaker Neo’s machine learning (ML) capabilities while using only a tenth of the memory.
• Edge Devices: Operating Models and Their Applications
Amazon SageMaker Edge Manager’s cloud-based design makes it simple to monitor and operate models operating on edge devices. SageMaker Edge Manager securely delivers data from devices to the cloud, where it is monitored, tagged, and maintained by specialists in order to constantly enhance model quality.
Text AWS to (415) 890-6431