Generative AI with Edge Computing
Edge computing is a decentralized computing paradigm where data processing occurs closer to the source of data generation, i.e., at the “edge” of the network. This contrasts with traditional cloud computing, offering advantages such as reduced latency locally to the device, improved bandwidth efficiency, and enhanced privacy. However, there are still some limitations to the computing power provided by each device. Given any physical limitations each device has, any calculations made locally may still struggle with a lack of resources.
The Integration of Generative AI in Edge Computing
Real-time Inference
The primary driver for applying Gen AI to empower IoT devices is to give the capability to make intelligent decisions based on real-time data analysis to the device. For instance, in a smart home environment, IoT devices can automatically adjust settings based on user behavior patterns without the need for constant connectivity to the cloud. Generative AI algorithms, when deployed at the edge, facilitate real-time inference on IoT devices to make reactions and decisions faster than if a device had to depend on a central server.
As an advanced AWS partner, we bring unparalleled expertise to architect, deploy, and optimize cloud solutions tailored to your unique needs.
Enhanced Privacy and Security
A flaw typically associated with IoT devices is the need to exchange information wirelessly with other devices in the network. Though not inherently bad, the data transfer still represents an opportunity to interrupt the data stream and either feed in bad data or steal important data. By processing data locally on IoT devices, generative AI contributes to enhanced privacy and security. Sensitive information can be processed and analyzed without being transmitted to external servers, mitigating the risks associated with data transfer and storage.
Bandwidth Optimization
Having all the calculations made locally to the device reduces the need for calculations at a single device and for data to be exchanged between devices, therefore providing lower latency. Generative AI algorithms can filter and process data at the edge, sending only relevant information to the cloud. In scenarios involving visual data, such as surveillance cameras or sensors, generative AI can dynamically generate content at the edge. This includes tasks like image recognition, enabling immediate responses to detected objects or anomalies without external processing. This optimization minimizes the amount of data that needs to be transmitted, reducing bandwidth usage and operational costs.
Additionally, this will provide IoT devices options to adapt and learn from their environment more naturally. Devices can evolve with the introduction of new data, improving their decision-making capabilities through continuous learning and data analysis. By extension, this will alleviate the need for centralized servers and constant updates. Without relying on centralized cloud servers, devices can make intelligent decisions autonomously.
Overcoming Future Challenges
There is only so much the application of Gen AI models can fix. The introduction of Gen AI to the Internet of Things could cause additional problems while remedying others. These are just limitations that developers will have to work around as they implement models at edge devices.
Model Updates
Ensuring that Gen AI models on IoT devices stay up-to-date poses a challenge. Along with providing new datasets accordingly, the software will need to be maintained on the devices on occasion. However, techniques like federated learning allow models to be trained collaboratively across devices without compromising data privacy.
Resource Constraints
Because of the aforementioned physical limitations, some networks might require from the network devices, there could be limits on what processing power the model has access to. These will just have to be constraints Gen AI models need to be optimized for before the deployment of an IoT device. Techniques such as model quantization and compression can address the challenge of limited computing power and storage.
Use Cases
Predictive Maintenance
Outside of analyzing data gathered by the device, models on IoT devices can analyze hardware data to predict equipment failures or maintenance needs. This predictive capability ensures timely interventions, reducing downtime and optimizing operational efficiency.
Healthcare Monitoring
IoT technology will continue to play a critical role in healthcare. Being able to continuously monitor vitals and the well-being of patients across an entire facility with limited staff numbers is invaluable. Devices equipped with generative AI can monitor patient data locally, providing real-time insights and triggering alerts for critical conditions. This facilitates timely medical interventions without compromising data security.
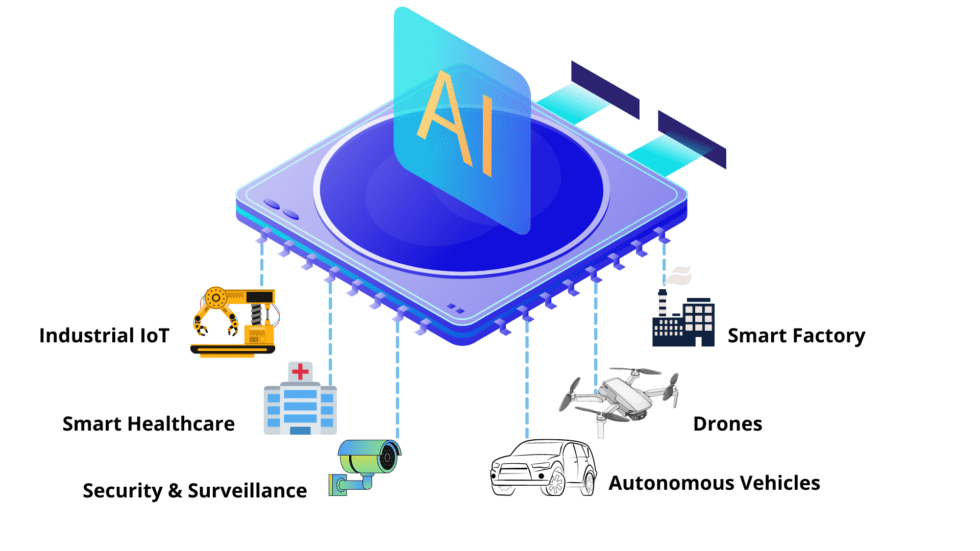
Autonomous Vehicles
Self-driving cars have been a long-anticipated technology. Gen AI could be the crucial component of autonomous vehicles by enabling on-board processing of sensor data and subsequent internal decision-making. This local processing ensures quick responses to dynamic road conditions, contributing to safer and more efficient transportation. Additionally, this will make hijacking automated vehicles more difficult for bad actors.
Future Advancements
As technology advances, the integration of generative AI with edge computing for IoT devices is expected to grow further. At this point, there is a limited scope to what we can apply it to, though that’s expected to change as the technology becomes more practical. Future trends may include more sophisticated edge devices, decentralized machine learning architectures, better security protecting these devices and their data, and increased collaboration among devices for collective decision-making.
Conclusion
Generative AI’s integration with edge computing represents a transformative shift in how IoT devices operate and make decisions. By enabling real-time inference, enhancing privacy and security, and empowering intelligent decision-making at the edge, generative AI contributes to the efficiency and autonomy of IoT ecosystems. As the synergy between generative AI and edge computing continues to evolve, it opens doors to new possibilities and applications, fostering a future where IoT devices are not just connected but also intelligent decision-makers in their own right.
Get Started Today!
At AllCode, our mission is to leverage our unique skillset and expertise to deliver innovative, top-tier software solutions that empower businesses to thrive in our world’s rapidly-evolving technological landscape.
Work with an expert. Work with AllCode
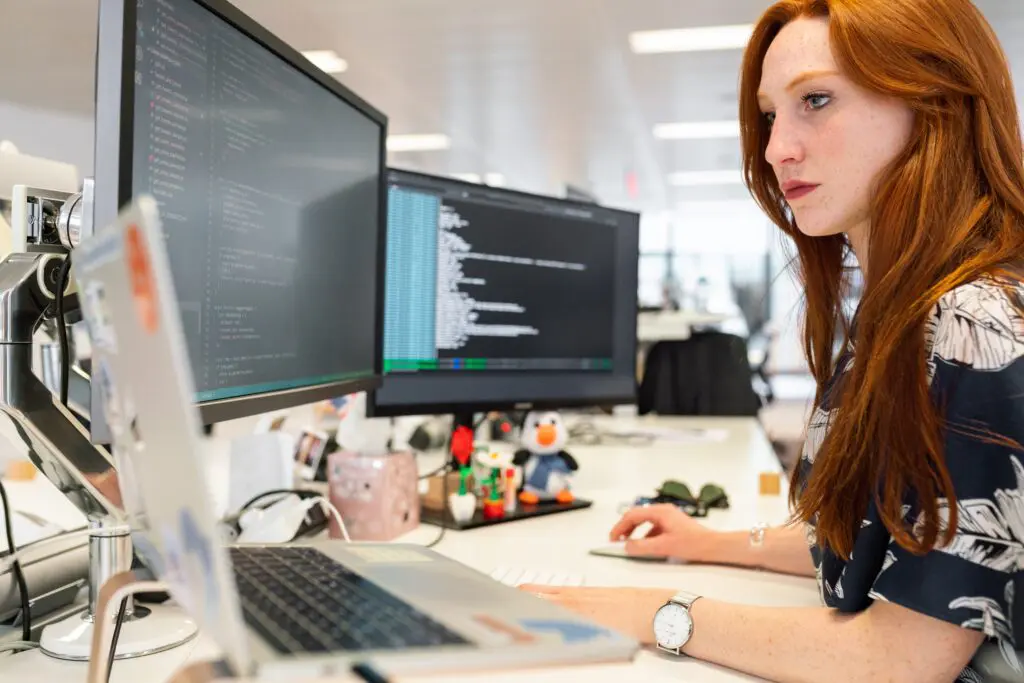