What is Generative AI and its Applications?
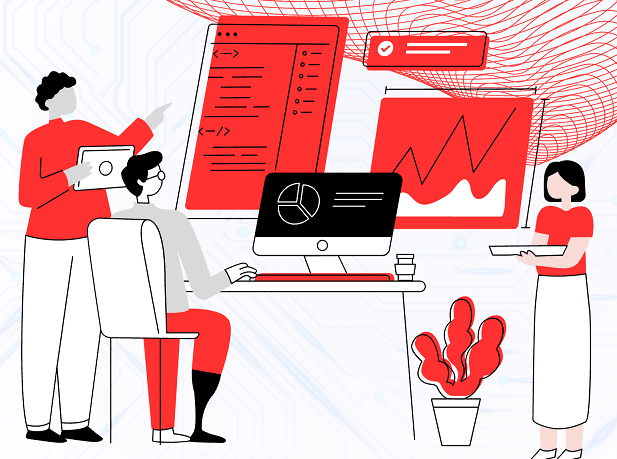
What is Generative AI and its Applications?
Generative AI is a type of technology that can synthesize a variety of content, including text, images, and audio. Though the technology necessary for generative AI has existed since the 60s, it hasn’t been until the mid 2010s that generative adversarial networks (GANs) have unlocked greater potential for this technology and have been applied towards more practical uses.
The main goal of generative AI is to generate creative and contextually relevant content, imitating the patterns in the data it was trained on. This primary goal of a generative AI model is achieved through its ability to process and reinterpret inputs like text, images, video, or audio, and transform them into a new output in the desired format.
Understanding what is Gen AI involves recognizing its capacity to combine multiple AI algorithms. A generative AI model starts with a prompt in the form of some existing text, imaging, video, audio, or any other physical input the AI can process. After interpreting the data provided, it will proceed to regurgitate the information into the implemented medium. Even after the most recent developments, how Gen AI works is still evolving as generative AI is still in its nascent stages and can at most produce the most basic outputs.
Need help on AWS?
AWS Partners, such as AllCode, are trusted and recommended by Amazon Web Services to help you deliver with confidence. AllCode employs the same mission-critical best practices and services that power Amazon’s monstrous ecommerce platform.
Model Types
Diffusion Models: Also known as denoising diffusion probabilistic models or DDPMs for short, diffusion models are generative models that determine vectors in latent space through a two-step process during training: forward diffusion and reverse diffusion. The forward diffusion process slowly adds random noise to training data. By comparison, the reverse process reverses the noise to reconstruct the data samples. Novel data can be generated by running the reverse denoising process starting from entirely random noise. Multiple iterations can then refine the logic the model uses. These models are popular examples of how Gen AI works to create detailed and unique content.
Variational Autoencoders: Variational Autoencoders or VAEs consist of two neural networks typically referred to as the encoder and decoder. When given an input, an encoder converts the input into a smaller, more dense representation of the data. This compressed representation preserves the information that’s needed for a decoder to reconstruct the original input data, while discarding any irrelevant information. The encoder and decoder work together to learn an efficient and simple latent data representation to imitate so it can be mapped through the decoder to generate new data. Though this model generally speaking can generate outputs faster than the Diffusion model, the outputs typically aren’t as refined.
Generative Adversarial Networks: First conceptualized in 2014, Generative Adversarial Networks or GANs were considered to be the most commonly used methodology of these three models before the recent success of diffusion models. GANs pit two neural networks against each other. The first network is a generator that generates new examples and a discriminator that learns to distinguish the generated content as either real or generated.
These two models are trained together incrementally to improve their ability to churn out content and to recognize whether the content is faked or not respectively. This is repeated until the generated content is determined to be indistinguishable from the original content. While the content produced is high-quality, the downside is that sample diversity tends to be weak, making GANs better for more specific data generation.
Making New Content Automatically
CloudContactAI (CCAI) has been a gradual project of adding more features to make it the most extensive and adaptive multi-media marketing program on the market. Most recently, we have begun implementing the email editor and email campaigns into the main features of what marketing tools users have access to. The email campaign maker is also going to be the first feature to incorporate generative AI examples, allowing users to create email templates effortlessly.
After opening the email builder to make a campaign from scratch or to make a template, users will be immediately presented with the option to build an email using a prompt. If the user doesn’t want to manually construct the email, they can type in a number of concepts as the basic prompt for the model to work with. After accepting the prompts, the generative AI will then write an abstract which the user can then continue to edit, implement other images, links, and features to the email, or accept as is. This process exemplifies the primary goal of a generative AI model: making content creation as seamless and efficient as possible. Upcoming updates will extend these capabilities to SMS marketing campaigns, further demonstrating what is Gen AI and its transformative potential in marketing.
Closing Thoughts
For the longest time, we have been looking for ways to integrate how Gen AI works into one of CCAI’s features. It’s a marketing platform where understanding customer trends is made easy and generating content is made to be as simple as possible, aligning perfectly with the main goal of generative AI.
If you’re interested in exploring what is Gen AI and how it can transform your marketing strategy or are eager to get started with CCAI’s generative AI beta, you can learn more and sign up on the CCAI website.